
Machine learning in a digital age: The future is now

Machine learning is one of those buzzwords that is often used, and sometimes abused…
Don’t get me wrong, it’s a super powerful tool in the age of data, but what does it really mean? And what does it actually deliver for digital and mobile marketers in particular?
In the most basic terms, machine learning is the application of Artificial Intelligence (AI) that enables a system to autonomously learn. AI attempts to simulate human intelligence, whereas machine learning pulls from past data and independently applies it to the performance of tasks. Over time, and with ever-increasing volumes of data, the machine is able to learn from previous tasks and improve the accuracy for decision making and predictions.
In digital marketing, machine learning algorithms can help understand trends in user behavior so that we can predict how valuable these users might be over time.
With the introduction of iOS 14 and its attribution limitations, the importance of predictions to enable app marketers to be equally effective rises significantly.
Machine learning also plays a key role in audience segmentation, personalization, media buying, creative optimization, and process automation.
In this blog we will explore the role and value of machine learning in digital marketing and specifically mobile app marketing, while providing specific use cases and relevant examples.
I predict you will benefit from this post, so let’s get started.
What’s inside
- Intelligent, data-driven analysis in digital marketing
- The advantages of machine learning in digital marketing
- Putting machine learning into action
- Predicting customer behavior
- Understanding insights from predictive analytics
- The future of ML
Intelligent, data-driven analysis in digital marketing
One of the key characteristics of digital marketing is the collection of data for campaign optimization.
Since all marketers collect data and are able to carry out some form of analysis, where they differ is in their ability to apply this data intelligently. That means investing in the right tools, the right infrastructure, and the right team (especially data scientists) to draw meaningful insights that actually drive business growth.
But data is only a means to an end, and basic analysis, such as CTRs, CTIs, and CPAs are no longer sufficient. Advanced tools such as predictive analytics and machine learning are needed to take data and decision-making to the next level.
One such advanced use lies in the opportunity to take this data and apply machine learning techniques to predict consumer trends, providing marketers with a powerful layer of intelligence that enables smarter decision-making.
The marriage of past actions to future predictions is a major reason why machine learning is such a game-changer for digital marketers. We’ll expand more on this point further down in the post.
The advantages of machine learning in digital marketing
There are many ways to leverage machine learning in digital marketing to make the most of your data:
Improved user segmentation
Digital marketers have the ability to learn about their customers over time by granularly measuring their activities.
A mobile app may have 200+ metrics available for measurement, but a typical marketer will probably measure a maximum of 25. A machine, on the other hand, is able to ingest all of that information in a matter of seconds and apply it to marketing efforts.
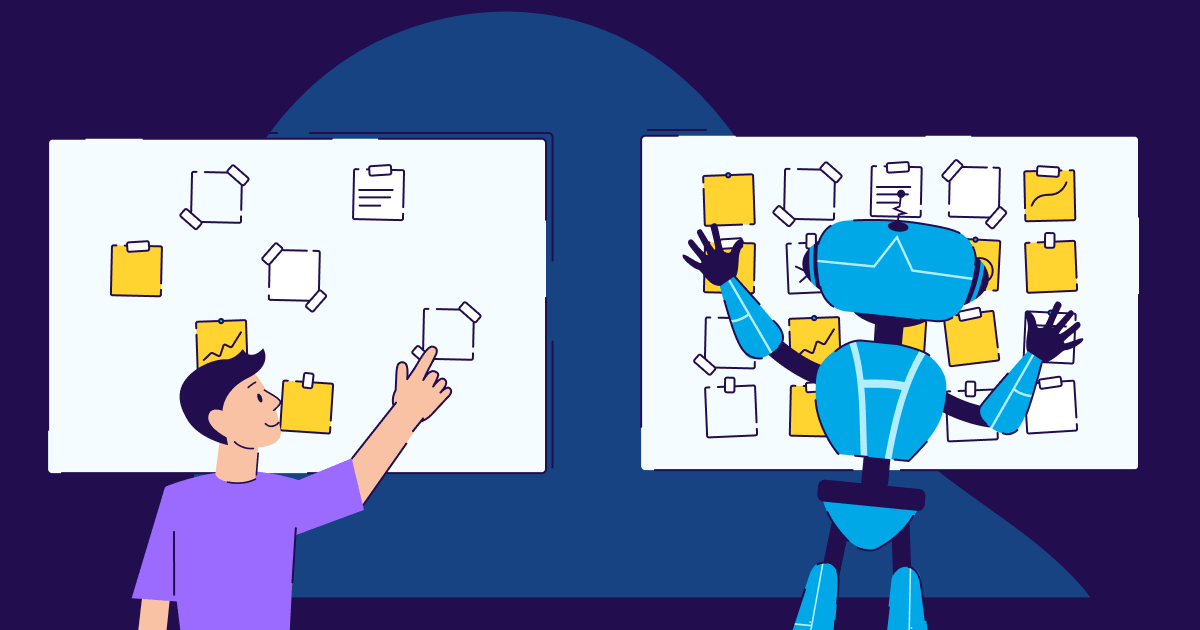
Let’s take an example:
An eCommerce app has tens of thousands of active users per day. With machine learning, a campaign manager will be able to predict, based on previous behaviors, which users are likely to purchase what next, and when.
Consider that power of knowledge. Armed with this info, the marketer could create a perfectly crafted campaign, meeting exactly the right users at exactly the right time.
Using indicators such as previous purchases, frequency of purchases, time of day, demographics, funnel progression, etc. the algorithm is able to cluster more general audiences and segment them into highly granular, mutually exclusive audiences for more effective targeting and messaging, and ultimately a better return on ad spend (ROAS).
A more personalized customer experience
With improved segmentation comes the ability to deliver a more personalized experience.
If you know where your customer is in the funnel then you can serve them with the ad that is best suited to their stage and their preferences.
When users are in the app, machine learning can use data such as search history and typical actions and pair it with behavioral data and search requests from similar users to suggest more products or services.
For example, a clothing app will likely know a user’s gender (assuming women view women’s clothing items and vice versa), previous purchases and how much they typically spend on an item. Coupled with information such as season and location, the algorithm brings all of these factors together and suggests items that can be used in re-engagement creatives for a more personalized experience.
Creative optimization
Knowing the optimal location, color, size, etc. of a CTA button or an image is something that we can experiment with using A/B testing. However, this is usually limited to one variable. Machine learning allows marketers to test multiple variables at any one time and offer insights on which was the most effective.
For example, a marketer may have dozens of campaigns running simultaneously, all with at least 10 different elements in each creative asset. Understanding which combination of elements, for example, colors, location on page, text, numbers of words in the CTA etc requires a machine to be able to analyze all of the possible combinations and offer insights into what delivers the best results.
Automation of processes
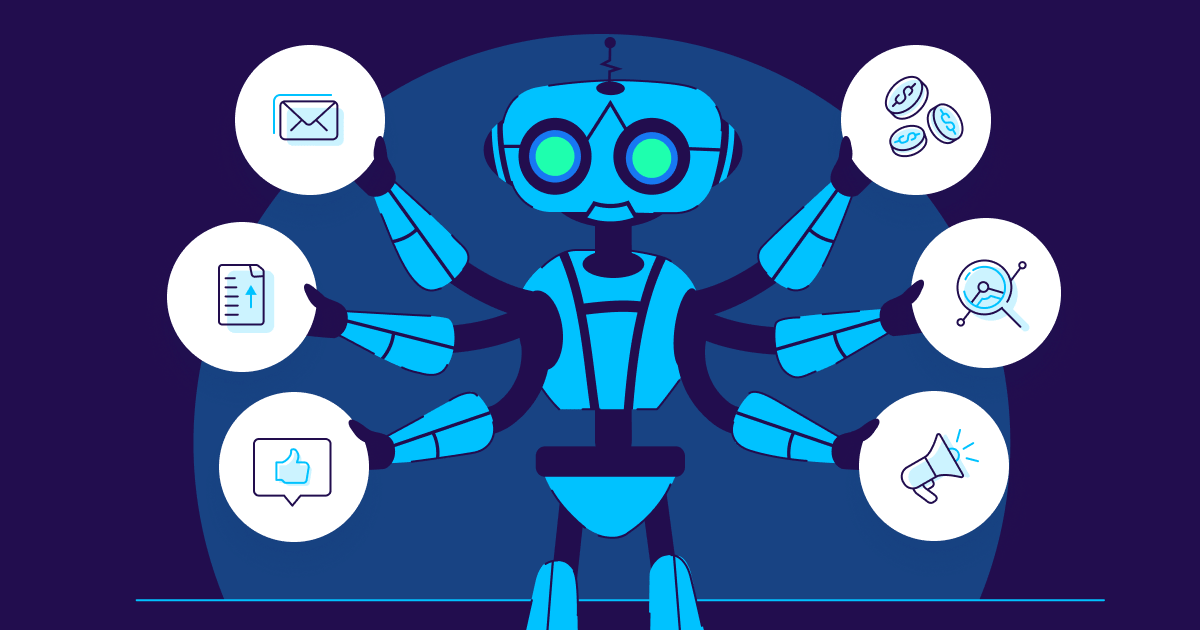
Machine learning is not just about the ability to better target users. At its core, it is about learning to perform tasks without human oversight. A marketing campaign is full of processes, many of which are hands-on, mundane, and repetitive.
By automating these tasks, marketers free up time to focus on issues which require human intervention. The net result is a more streamlined and efficient process, fewer human errors, and more capacity for marketers to deal with complex and strategic tasks.
For example, campaign managers often run dozens of campaigns at any given time. They typically start their day by looking at a dashboard of data and assess which campaigns are running well and which are not, and adjust their marketing efforts accordingly. What they are relying on to optimize their campaigns is mostly based on their own experience and intuition.
Automation achieved through machine learning, however, can be used to better optimize campaigns. Algorithms are able to rapidly and accurately process and analyze campaign data and trigger notifications when certain trends or unusual spikes occur. These alerts are essentially insights with which the campaign manager can decide whether to stop, adjust, or double-down on their marketing efforts.
Putting machine learning into action
The impact of machine learning goes far beyond these advantages, and is in fact a major influence to mobile marketing efforts as a whole. There are numerous examples and use cases but let’s explore some of the main ones.
Ad fraud
Fraud has and will continue to plague the web and the app world. It is estimated that $1.6 billion were exposed to app install fraud alone in H1 of 2020 (the amount of ad spend that would have been lost to fraud had there not been any protection in place). Outsmarting the fraudsters is a never-ending challenge.
Machine learning is a vital tool to effectively and efficiently combat fraud by analyzing common user behavioral trends using these insights to highlight any activity that might deviate from these trends.
While no human can simultaneously compare data trends across dozens of device indicators, engagement and timing trends and spot the odd bird, a machine can do so in a matter of milliseconds.
Moreover, the larger the available data set is, the faster the machine can identify existing and new fraud trends as they emerge. The machine can block them with greater statistical significance and confidence.
Chatbots
Delivering a superior customer service is also a major goal for most businesses, and app owners are no different. Chatbots are computer programs that can engage people in human-like conversations, and the most advanced versions are driven by machine learning. In their most basic form they offer the opportunity for 24/7 customer service.
More advanced machine learning driven bots can help customers complete purchases and answer FAQs. You can reduce your customer support costs, having the bots escalate any queries the algorithm can’t answer.
Autodesk, a 3D computer-aided design company used their bot, AVA for precisely this purpose. Their bot responds to simple tier 1 queries such as address changes, payment issues, and login issues anytime of the day or night. Their bot service improved their response times by 99% and dropped the cost per query from $15-$200 (human customer service agents) to $1. The bot has 40+ conversations it can effectively resolve and all other issues are escalated.

Taking it a step further, digital marketers can enhance their interactions with customers by using data from chatbots to deliver a more personalized experience to their users.
For example, using the information generated during a conversation with a chatbot to inform a remarketing campaign.
Dynamic pricing
Travel apps want to know they are pricing their products and services at the market rate. For example one hotel booking app will not want to price the same hotel room much higher or lower than their competition. Dynamic pricing uses machine learning to monitor the web and ensures that the price you are quoting is the correct market value at that moment in time.
Additionally, it can also predict which users may be close to purchasing and offer timely discounts. Such discounts can be limited to those who are close to converting, but require an extra incentive. This way price cuts can be offered in marketing campaigns on a limited basis and to those who would not otherwise convert.
User acquisition
Media sources, with their vast scale of data, are able to leverage machine learning in user acquisition campaigns for app marketing. For marketers, it’s important to understand how your media partners are leveraging machine learning in an effort to optimize your campaigns.
Facebook developed a tool called Automated App Ads (AAA) which, by using machine learning, allows marketers to test audiences in a quick and efficient manner. They can test keywords or creatives and analyze the results to see which ones received the best conversion rates.
The Google App Campaigns (AC) product uses machine learning to analyze hundreds of signals in real-time so that your app appears in front of the user most likely to convert. AC also uses this technology to optimize bids across Google’s properties. It does this by taking campaign types, in-app events, and in-app conversion values and incorporating them with other variables such as copy, creative, budget, KPIs, and a bid.
Google then takes all of this information and, using machine learning, tests it across its properties including, search, Google Play, Discover, the Google Display Network, and increasingly, YouTube, serving the ads which are performing the best.
Ad networks, demand-side platforms, and exchanges, as well as mediation platforms and other sell-side players also leverage machine learning in programmatic campaigns, particularly ones that involve real time bidding.
Understanding customer behavior
Finally, and crucially for app marketers, machine learning is an excellent way to understand customer behavior. We mentioned it briefly before but the ability to predict how a customer is likely to behave based on their previous interactions is an incredibly useful tool to marketers.
Let’s explore this concept a little further.
Predicting customer behavior
App marketers have a sea of data available to them, not all of which is utilized. However, with machine learning they can leverage past performance trend analysis to construct a solid prediction for future trends and even a customer’s next action.
Hypothetically, a machine can know, for example, that a user who completes a tutorial and passes three levels of a game in their first day post install is more likely to purchase tokens. Having this knowledge can help app marketers decide early on whether to optimize a campaign, kill it, or increase spend.
We have mentioned that the more data the machine has the better the assumptions it can make. Only once there is sufficient data and a certain confidence level is reached can this assumption be considered a prediction. It is important that the prediction is delivered at the right moment so that the marketer can act on the insight before they lose the opportunity to create value from it. Too little data and the assumption may be incorrect, wait too long and you may have missed the moment to optimize.
Therefore tight integration with data-rich dashboards, such as in-app events, which best reflect a customer’s LTV pattern will enable a prediction algorithm to deliver faster, more accurate results.
Your attribution partner is perfectly positioned to provide such data and as such helps build the picture of how well a campaign is performing. Having an attribution provider that has machine learning built into its system will enhance this already smart solution.
Think of your attribution data as the infrastructure to your marketing campaigns. Your attribution dashboards are home to vast quantities of data and are constantly acclimating more. The ability to predict what a user, or a group of users, may do next is a significant advantage when assigning budgets and resources.
Understanding insights from predictive analytics
Marketing analytics help to deconstruct the data by highlighting trends and delivering insights so marketers can gage which users are the most valuable and which channels are the most profitable.
The process of understanding the data and generating insights can take a number of weeks, or even months.
Predictive analytics help to cut the campaign learning period by using existing integrations to provide an accurate campaign LTV prediction.
By leveraging machine learning and understanding the existing user-level information, predictive analytics could feasibly deliver a campaign score within days of its launch, telling the marketer how successful it is likely to be. With this information, the marketer can either cut the campaign, optimize where required, or double down giving them the ability to make a fast pause-boost-optimize decision.
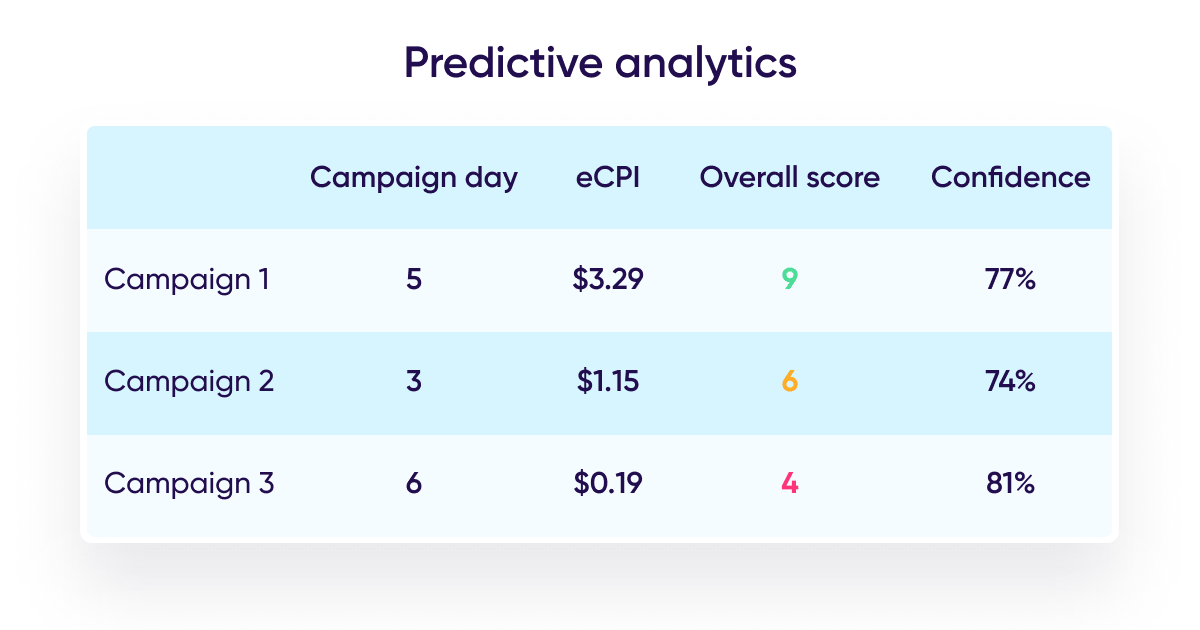
The SKAdNetwork challenge
The challenge comes with the introduction of iOS 14 and the use of the SKAdNetwork which aims to create a more privacy-centric environment, and limit the measurement of user-level data in the iOS ecosystem.
For Android and those that opt-in to IDFA measurement, traditional attribution is still available. Data is available much faster and on an on-going basis, so marketers are able to optimize without necessarily using predictions (though using predictions is always advised and used by the savviest marketers).
However, in iOS 14, the quantity of data is severely limited.
The SKAdNetwork allows only one postback per campaign and so there is a limit not only on the volume of data, but also the time in which the marketer has to be able to make decisions on whether a campaign is likely to be successful.
When you compare the capabilities of traditional attribution models to what is possible in the SKAdNetwork you see how vitally important machine learning is for app marketing. Machine learning algorithms can quickly learn and most importantly, predict, which campaigns are likely to deliver the most valuable customers.
Beyond the limit of one postback per campaign, there are other limitations to the SKAdNetwork including a lack of real-time data, no ROI or LTV data as it mostly measures installs, and a lack of granularity as only campaign level data is available (and that is limited to 100 campaigns).
Additionally, there is a 24-hour, and sometimes longer, post-back delay and there is no re-engagement attribution support.
So the question really is, in this post-iOS 14, SKAdNetwork reality, how can you deliver relevant advertising without knowing what action each user is performing?
Enter machine learning, which will enable ad networks to continue to provide value without having to create intrusive user profiles.
One way can be by using statistical estimations and previous data to predict future actions. Marketers can also run experiments using non-personalized parameters such as the contextual signals mentioned earlier and train their machine learning models on what was successful and what wasn’t. The results are then applied to future campaigns and further refined as more data is collected.
The future of ML is now
Machine learning isn’t going anywhere. In fact, its abilities are only getting stronger. There is a concern that these algorithms will take over some roles, and this may be the case, but they will likely be repetitive, time-intensive, mundane tasks that marketers don’t enjoy. The flip side of this is that it will free up resources and time to be used on more critical tasks which require human intervention.
One example of this are the creatives. A machine may be able to make keyword predictions but it can’t develop a nuanced idea or design the creatives to go alongside a campaign. There are some aspects of digital marketing where the human touch is still vital and creatives are an example of this.
With the introduction of iOS 14 machine learning will be an extremely important tool for marketers who will need to demonstrate that data driven marketing can still thrive in the age of privacy.
Key takeaways
Although the application of machine learning within mobile marketing is still in its infancy, it is certainly here to stay. AI and machine learning have already made a significant impact on marketing analytics and we predict this is only the beginning of the journey.
Let’s summarize what we have learned:
- Machine learning enables smart decision making using historical data to predict future trends
- There are many examples of how machine learning can be applied to marketing efforts including:
- Audience segmentation
- Combating fraud
- Delivering a superior customer experience
- Improved user acquisition
- Ability to dynamically price products
- Machine learning can help us understand customer behavior in less time and enable marketers to predict future trends and actions faster and more accurately
- Machine learning will be a critical tool in the post-iOS 14 era as the quantity of data and the time marketers have decide the value of a campaign are severely limited. The ability to predict trends based on the data available will therefore be critical to marketers going forward.
Finally, the more data we have, the smarter and more precise the predictions become.
Of course there will be some aspects of mobile marketing that machines will never be able to replicate, but the more we embrace the capabilities of machine learning, the smarter we as marketers become.